
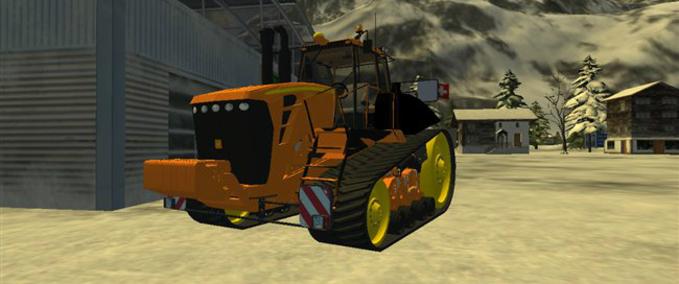
These models have become useful tools for flood risk assessment and management, especially in regions where little local hazard information is available. The growing worldwide impact of flood events has motivated the development and application of global flood hazard models (GFHMs). performance tends to be highest in humid and lowest in arid catchments, and catchment size (Salinas et al 2013), and that regional regressions that divide a domain into subregions and apply regression models separately always perform better than ek et al 2014). Studies comparing RFFA methods have also shown that performance of predicting flood magnitudes tends to have a correlation with climatological factors, i.e. Previous studies comparing GHMs have shown that there is variability in performance of model simulations of peak flows in space (Towner et al 2019), the effect of routing scheme implemented can be critical in the simulation of peak discharge magnitudes (Zhao et al 2017), that in many environments the uncertainty from input data quality is of a similar magnitude of model structure uncertainty (Marthews et al 2020), and there is significant uncertainty in global scale hydrological modelling from precipitation data errors (Sperna Weiland et al 2015). However, this previous work has focused on the deterministic final outputs from a number of GFHMs which has not allowed for direct attribution of the differences between these models to any specific factors. Maps (e-h) show the corresponding spatial distributions of log 10 (α X,j ) values for each variable, with the colour scales corresponding to the vertical axis on scatter plot (a).
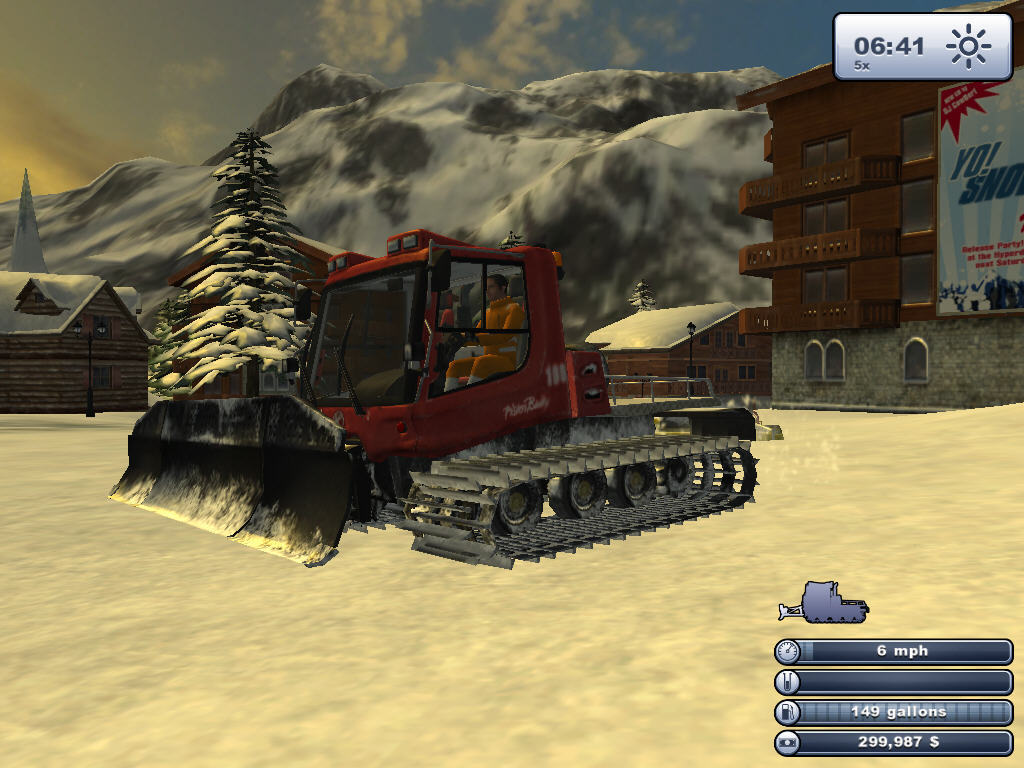
Linear regression lines for each latitudinal zone indicate the trend as precipitation increases within each zone (all regressions were significant at the 1 % level), although, n.b., we do not contend in any way that the distribution of points shown is linear: these lines simply indicate a trend that is not clear to the eye from the envelopes displayed (which do not show the complete point cloud). Because of the density of overlapping points, only the envelope of points for each latitudinal zone is shown and the points with the highest uncertainty (uncertainty DIU ≥ (2/3) Points on the scatter plots are coloured according to latitudinal zones (Fig. 1) (log 10 (α X,j ) 0 indicates uncertainty in the predicted variable X is greater), where X is evapotranspiration (a, c, e, f) or runoff (b, d, g, h) and j refers to either high extremes (a, b, e, g) or low extremes (c, d, f, h). Values of log 10 (α X,j ), where α X,j is the scaled data uncertainty in variable X (Eq. Our results are important for highlighting the relative robustness of satellite products in the context of land surface simulations of extreme events and identifying areas where improvements may be made in the consistency of simulation models. We also found that high data and model uncertainty points for both ET lows and runoff lows were disproportionately concentrated in the equatorial and southern tropics. Uncertainty in predictions of evapotranspiration lows (drought) in dry environments was especially high, indicating that these circumstances are a weak point in current modelling system approaches. In percentage terms, the increase in uncertainty was most often less than the magnitude of the input data uncertainty, but of comparable magnitude in many environments.
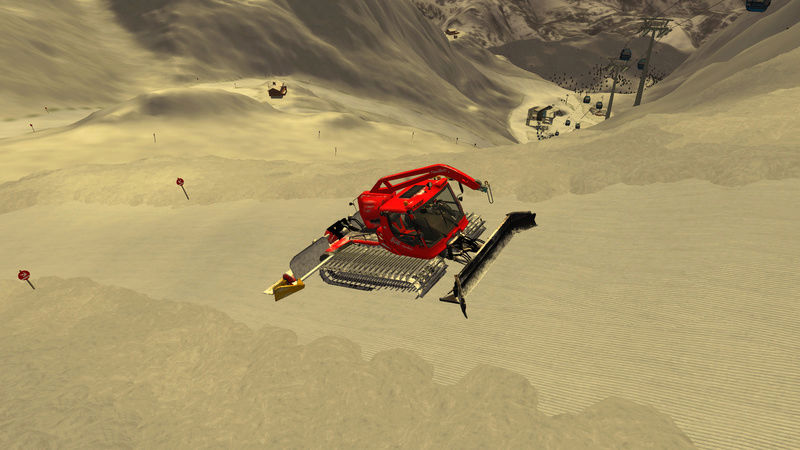
We found in general that model simulations acted to augment uncertainty rather than reduce it. Uncertainty was apportioned between the model used (model skill) and also the satellite-based precipitation products used to drive the simulations (forcing data variability) for extreme values of precipitation, surface runoff and evaporation. We evaluated the performance of five state-of-the-art global hydrological and land surface models in the context of modelling extreme conditions (drought and flood). Knowledge of how uncertainty propagates through a hydrological land surface modelling sequence is of crucial importance in the identification and characterisation of system weaknesses in the prediction of droughts and floods at global scale.
